A survey by Gartner Research found that poor data quality
costs companies an average $8 million per year. In a different study published
by The Data Warehousing Institute more than 50% of companies experience
customer dissatisfaction and cost problems due to poor data.
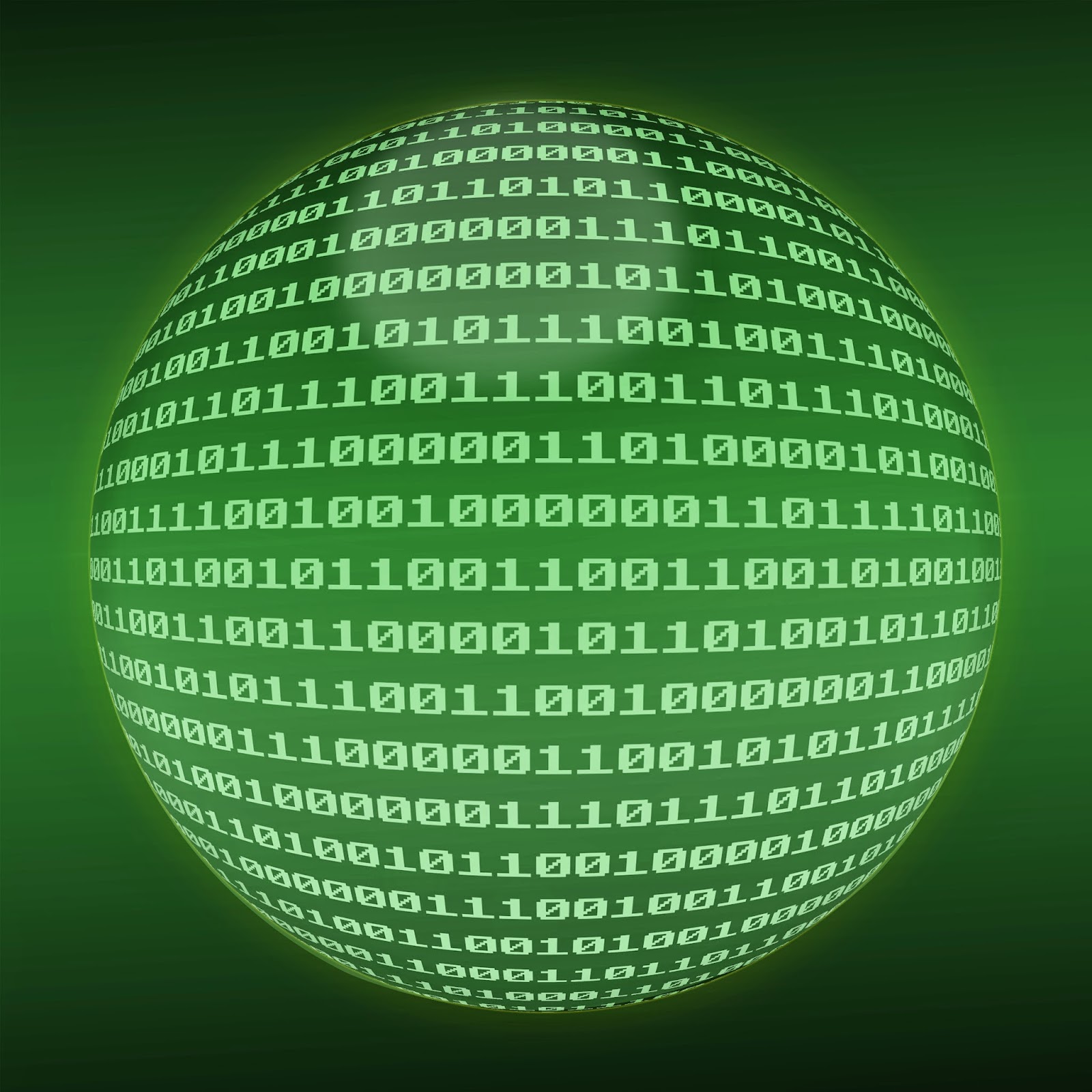
It’s pretty obvious that the main reason for the failure to meet Business Intelligence expectations is probably data quality; reinforcing the proverbial GIGO principle.
This is only getting worse considering that Aberdeen Group
estimates the amount of corporate data companies need to digest is growing at 56% annual rate, coming from an average 15 unique data sources.
It’s clear that today’s Business Intelligence &
Analytics software capability is light-years ahead of the semantic quality and
structure of the data. After years and millions of dollars spent in BI
deployment, folks in areas like Marketing, Sales or Strategic Management feel
like they are drowning in an ocean of data and yet thirsty for the Strategic
Knowledge they need to grow the business.
It’s common to see state of the art Business Intelligence
applications that cannot deliver strategic analysis or direction until multiple
analysts download the data into spreadsheets, manipulate, clean, fix and
structure the data manually, for hours or days at a time.
Many folks recognize the transaction data has plenty of
errors, at least at the customer, product line, brand, market and segment
level, but they never get fixed and, even worse, they populate the BI systems.
This is a classic case of cultural miscommunication.
Marketing and Sales people think this is an IT problem and expect the data
warehouse analysts to fix it, while IT thinks it’s a business problem and
expects Sales or Marketing to take action. The result is that data seldom gets
corrected, people give-up, and running raw or bad data through sophisticated BI
systems and dashboards becomes the norm.
After a while, Sales, Marketing and Management won’t trust
the data, will not see the value of BI, will quit using the system and continue
making decisions based on intuition.
When Analysts or Power users need a quick answer about
market share, profitability or growth of a particular segment, product line,
customer or competitor it takes days to go through the analyst’s manual
process. This consists of running the right queries, exporting them to
Microsoft Excel, manually cleansing data errors, adding look-ups from external
data sources, and finally creating pivot tables to find the right answers.
Even worse, they have to go through the entire process over
and over again every time they need a progress update, either the following
week or at month-end, quarter-end or year-end for each one of the business
units and markets they serve. This not
only is an inefficient process but fixing data based on analysts’ personal
assumptions leads to undesirable multiple silos and different versions of the
truth.
As a result, it’s common for people to spend a large portion
of team meetings arguing whose data is correct instead of focusing on the
critical issues. The numbers generated by Finance do not agree with the
analysis performed by Marketing or the explanations provided by Sales. They
need a single version of the truth, but different folks run different queries, made
different data cleansing assumptions and customized their spreadsheets based on
different metrics.
A Simple Solution that Works:
While IT may own the physical aspects of the data (storage,
security, format, connectivity, etc.), the Business (e.g. Marketing &
Sales) must own its strategic meaning and be committed to auditing and
maintaining its high quality.
Proficient analysts who spend most of their time cleansing
the data constantly after downloading it to spreadsheets every time they need a
report, need to change and become Data Stewards that cleanse and structure the
transaction data using external market intelligence BEFORE it enters the Data
Warehouse or BI systems. They need to maintain, update, format and upload their
data corrections on a weekly or monthly basis.
This small process change requires a committed partnership
between IT and the Business Units but truly makes a difference because it
breaks the Business Intelligence vs. Data Quality vicious cycle.
When the data makes sense to BI users they start to
recognize their business models in their dashboards, develop trust in the data
and become better users. They’ll soon find additional data issues and take the
initiative to get them promptly corrected. In a few weeks there will be no more
serious data quality issues as this process becomes a virtuous cycle where the
BI utilization rate grows giving the company an analytical competitive edge.
No comments:
Post a Comment